If the last pandemic has taught us anything, it is that developing a new treatment in such a short period of time is achievable. This is true not only in the development of vaccines, but antibiotics, immune regulators, and chemotherapy drugs are also required to go through an extensive process of research and development.
The discovery of a new drug or treatment is seldom accidental, but it is rather an intentional result that comes from the need to find a solution to an existing problem.
However, something that is done quickly can have drawbacks in terms of quality. This invariably stems from the level of accuracy and thoroughness that the assessment of the different variables that an experiment needs.
Thoroughness and accuracy over a certain period of time condition the results of drug development, regardless of the economical resources poured into it. In this scenario, the use of artificial intelligence (AI) in the discovery, development, and data analysis pertaining to drug development facilitates the formulation of experimental models, automates specific facets of the experiment’s control, and improves pattern finding in data analysis.
Discovery
Artificial intelligence increases the efficiency of every step of the drug discovery process. A higher efficiency implies lower costs, which also impacts the research in a positive manner. An example of how this software helps the process move along is also an example of how research and development itself is changing: simulations.
Determining the correct molecular structure of a given substance requires a careful design coupled with experimental models that need to be continuously tested. That design – traditionally done only by humans – can take advantage of an AI that “designs” a molecule based on human input and even simulates the interactions it would have with other molecules in the same environment. This helpful feature of using an AI solution is based on its capacity to analyze large amounts of data.
This can also be applied to the pattern identification of an illness by suggesting which drug would be the best candidate to employ as a treatment. Making use of these new tools allows companies to be at the forefront of the development wave. In this context, big pharmaceutical entities like Bayer and Pfizer are using these new informatic solutions in their own drug fabrication process.
Experimentation
Indeed, costs impact research directly in the experimentation phase of drug development. If we include clinical trials, then a very small percentage of these substances will be successful in their application and subsequent approval to be commercialized. Individual drug research, development, and production costs vary between $1 to $2 billion dollars, including clinical trials. In this landscape, the use of artificial intelligence is a key aspect in the birth of a new drug.
This software tool can be employed in the automation of different apparatuses – like centrifuges, homogenizers, and bioreactor cell culture – and different phases of an experiment. In turn, it enhances the quality of control and information gathering. Even more essential, the use of machine learning leads to a better experiment dynamic and efficacy, lowering the waste of resources.
An example of this is setting up an experiment and a simulation of said experiment in parallel. In this setup, the simulation receives detailed data from the processes that take place in the real experiment. The strength of this application resides in the algorithm that controls both the experiment and the simulation. The ultimate goal is to predict the outcome of processes that are yet to take place in the real experiment, optimizing resources and increasing efficiency.
Diagnosis
A newly developed drug is useful only when paired with an accurate diagnosis. AI-assisted diagnosis entails the use of electronic records for high quantities of patient data. This information consists of clinical histories, laboratory tests, images, and previous diagnoses. The analysis of this influx of information is done in a short period of time and opens the door to a more personalized treatment for each case. This can even be done in real-time.
Machine learning algorithms can be applied to real-time monitoring of a patient through intelligent glasses, wristwatches, or any other type of wearable appliance. This method isn’t only limited to those people who are in the active process of getting a diagnosis, either. People that are otherwise healthy can benefit from these devices and their software – and more importantly, metabolic, neurologic, and cardiological conditions can be detected and receive early treatment thanks to the use of these tools.
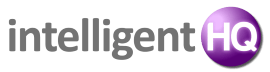
Founder Dinis Guarda
IntelligentHQ Your New Business Network.
IntelligentHQ is a Business network and an expert source for finance, capital markets and intelligence for thousands of global business professionals, startups, and companies.
We exist at the point of intersection between technology, social media, finance and innovation.
IntelligentHQ leverages innovation and scale of social digital technology, analytics, news, and distribution to create an unparalleled, full digital medium and social business networks spectrum.
IntelligentHQ is working hard, to become a trusted, and indispensable source of business news and analytics, within financial services and its associated supply chains and ecosystems