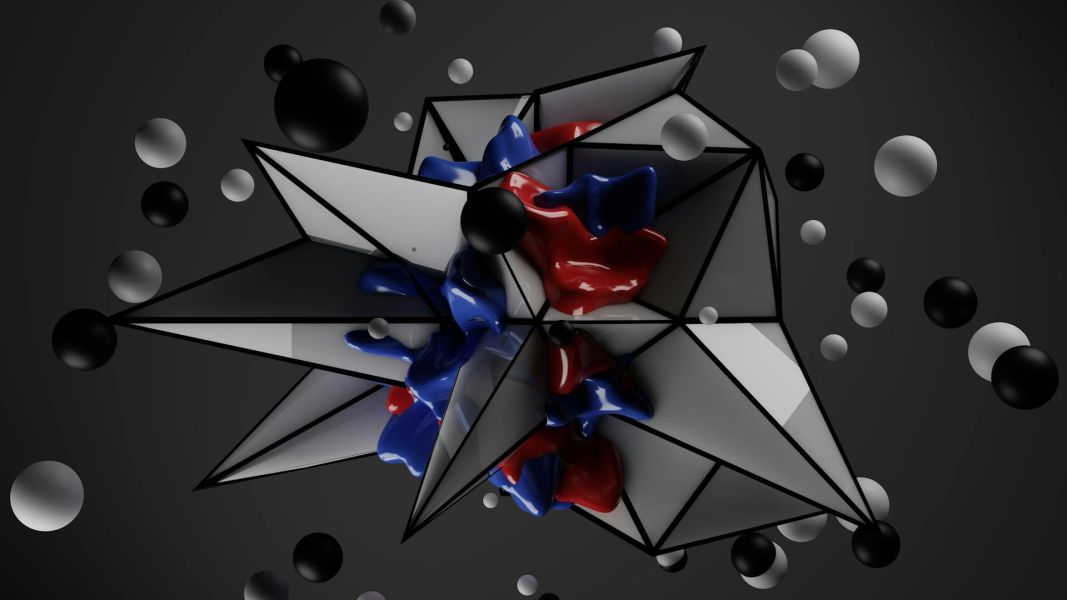
High-quality labeled data forms the backbone of AI training, though annotation expenses can grow quickly. Whether you’re working with AI data labeling for images, text, or audio, finding the right balance between cost and accuracy is key.
This guide provides actionable advice for improving your budget efficiency. You can use an image labeling tool, outsource to data annotation websites, or improve your labeling process. You’ll discover methods to lower expenses without compromising quality.
Understanding Data Annotation Costs
The cost of data labeling can vary widely based on project size, complexity, and approach. Knowing what influences pricing helps you allocate resources efficiently and avoid overspending.
What Affects the Cost?
Several factors impact AI data labeling costs:
Data size and complexity
More data means higher costs. Complex tasks, like 3D object detection or sentiment analysis, take more time and effort.
Annotation type
Simple bounding boxes are cheaper than detailed segmentation or NLP tasks.
Quality requirements
Projects needing multiple reviewers or strict validation cost more.
Manual vs. AI-assisted annotation
Using an annotation AI tool can save money, but some data still needs human review.
In-House vs. Outsourced Annotation
Each option has its own trade-offs:
Factor | In-House Team | Outsourced Services |
Upfront Cost | High (hiring, tools, training) | Lower (pay per project) |
Scalability | Limited by team size | Easily scales up or down |
Quality Control | Full control | Depends on provider |
Flexibility | Best for long-term needs | Ideal for one-time projects |
Many businesses mix both — outsourcing bulk work to an expert data annotation company while keeping a small internal team for review.
Strategies to Reduce Annotation Costs Without Sacrificing Quality
Cutting costs doesn’t have to mean lower-quality data. By optimizing your workflow and using smart strategies, you can save money while maintaining accuracy.
Prioritize Data Quality Over Quantity
More data doesn’t always mean better results. Labeling massive datasets can be costly and time-consuming, especially if much of the data isn’t useful. Instead:
Focus on high-impact data
Identify the most valuable samples for training.
Clean and preprocess data
Remove duplicates and irrelevant entries before annotation.
Use representative samples
A smaller, meticulously curated dataset achieves better results than a large, chaotic one.
Leverage Active Learning to Cut Costs
Active learning helps models request human input only when necessary, reducing workload. Key strategies include:
Prioritizing uncertain samples
The model flags data it’s unsure about for human review.
Iterative labeling
As the model improves, it needs fewer manual annotations.
Using AI-assisted tools
Many data labeling tools integrate active learning to speed up annotation.
Use Pre-Annotated and Public Datasets
Building datasets from scratch is expensive. Instead, consider:
- Pre-annotated datasets. Some providers offer industry-specific labeled data.
- Publicly available datasets. Many open-source datasets can supplement your project.
- Hybrid datasets. Combine public and proprietary data to lower costs.
Be mindful of licensing restrictions when using third-party data.
Optimize Workforce Costs
Annotation costs vary based on who does the work. Ways to save:
- Match skill level to task. Use domain experts only when necessary.
- Crowdsourcing vs. managed services. Crowdsourcing is cheaper but requires quality control.
- Train annotators. A well-trained team reduces errors and rework.
Tools and Technologies to Streamline Data Annotation
The right tools significantly ease manual tasks and bring down annotation spending. Choosing the proper software combined with AI enhances the speed and efficiency of the process.
Choosing the Right Software
The right data labeling tool can reduce manual effort and cut costs. Key features to look for:
- Automation capabilities. AI-assisted tagging speeds up the process.
- Collaboration tools. Multiple users can work on a dataset at the same time.
- Quality control features. Built-in validation helps reduce errors.
- Integration options. Works smoothly with your existing AI pipeline.
Open-source tools are free but can require both installation efforts and regular maintenance. Paid solutions offer more features and support.
AI-Assisted Annotation: When to Use It
AI-powered annotation tools manage repetitive tasks effectively, though human supervision remains essential. Best use cases include:
Pre-labeling | Object detection | Text and speech processing |
AI generates initial labels for human reviewers to refine. | AI speeds up bounding box and segmentation tasks. | NLP models can automate entity recognition. |
AI assistance works best when paired with manual verification to ensure accuracy.
Measuring ROI and Long-Term Cost Management
Keeping annotation costs under control requires continuous monitoring. Tracking key metrics and designing for scalability secures long-term efficiency without wasteful spending.
Tracking Costs and Performance
Understanding where your budget goes helps control spending. Key metrics to track:
- Cost per labeled data point. Helps identify expensive annotation types.
- Annotation speed. Measures efficiency and potential bottlenecks.
- Error rate. High correction rates indicate training gaps or quality issues.
- Reusability of data. Well-annotated data can serve multiple models, reducing future costs.
Using these metrics, you can refine processes, cut unnecessary expenses, and improve AI data labeling workflows.
Scaling Efficiently Without Overspending
As your dataset grows, so do costs. Keep spending under control by:
- Batch processing. Label only what’s needed at each stage, avoiding upfront over-annotation.
- Reusing existing data. Previously annotated data can train new models.
- Budgeting for continuous annotation. AI models improve with fresh data, so plan for ongoing annotation needs.
A structured approach to data annotation websites and tools ensures cost-effective scaling.
Common Mistakes That Drive Up Costs
Steering clear of frequent pitfalls saves time and money while maintaining high-quality labeled data. Here are costly mistakes to spot:
Over-labeling unnecessary data
Not all data points need annotation. Prioritize only the most valuable samples.
Skipping data cleaning
Poor-quality or duplicate data increases annotation time and cost. Preprocessing your dataset reduces waste.
Ignoring quality control
Inconsistent labeling leads to rework. A well-defined validation process minimizes errors.
Choosing the wrong method
Some tasks can be automated, while others require human input. Using the right approach prevents overspending.
Avoiding these missteps ensures you get the best value from your annotation funds without compromising precision.
To Sum Up
Optimizing your AI data labeling budget is about working smarter, not just cutting costs. Focusing on high-quality data and using the right tools can cut costs. Automation helps maintain accuracy, too.
A smart strategy mixes manual and AI help for annotation. It tracks performance metrics and plans for growth. This keeps things efficient over time. Careful budgeting and the right tools make high-quality annotation both affordable and sustainable.
Founder Dinis Guarda
IntelligentHQ Your New Business Network.
IntelligentHQ is a Business network and an expert source for finance, capital markets and intelligence for thousands of global business professionals, startups, and companies.
We exist at the point of intersection between technology, social media, finance and innovation.
IntelligentHQ leverages innovation and scale of social digital technology, analytics, news, and distribution to create an unparalleled, full digital medium and social business networks spectrum.
IntelligentHQ is working hard, to become a trusted, and indispensable source of business news and analytics, within financial services and its associated supply chains and ecosystems